Optimizing ChatGPT Performance
Optimizing ChatGPT Performance
The Unexpected Tool for Troubleshooting AI
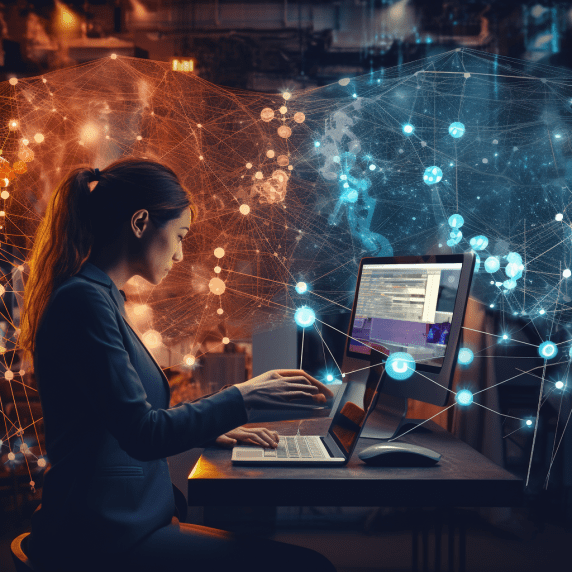
Consider this scenario - you’re an expert craftsman armed with a groundbreaking new tool. It promises unparalleled efficiency and breakthroughs, but at times, the tool doesn’t quite work as you expected. The outputs seem off, and you can't quite figure out why.
Now replace "craftsman" with "professional," and "tool" with "Artificial Intelligence (AI)."
Sounds familiar?
Even with the best AI tools like ChatGPT, things can go awry. Maybe your outputs are subpar, or your results unpredictable. The question is, how do we best troubleshoot this?
Interstingly enough, the answer could lie in a time-tested tool you may already know well - Thomas Gilbert's Behavior Engineering Model (BEM).
The Behavior Engineering Model
The Behavior Engineering Model was developed by Thomas F. Gilbert, a pioneer in the field of performance improvement. Originally devised to identify root causes of human performance gaps, Gilbert’s model outlines six critical factors that influence behavior - data, instruments, incentives, knowledge, capacity, and motives.
But here's an interesting twist. This model, originally designed for humans, offers surprising value for troubleshooting our collaborations with AI systems.
Data: A Bridge for Better Communication
In the Behavior Engineering Model, 'data' represents the expectations and information needed to perform a task efficiently. In our human-AI interactions, data equates to the prompts we provide to ChatGPT. Thoughtfully crafted prompts are like constructing a bridge, providing a clear path for AI to reach the outcomes we desire.
Consider a situation where you're using ChatGPT to create a series of emails for an upcoming product launch. The AI’s output will be vastly improved if you provide a detailed prompt that includes the product’s unique selling points, target audience, and key message you want to convey. The more specific your input, the more likely the AI will generate content that hits the mark.
Data also encompasses feedback, something AI also needs from us. If a response isn't aligned with our expectations, providing feedback allows ChatGPT to make adjustments and improve the initial output.
Instruments: Navigating Technical Challenges
Instruments, as defined by Gilbert, involve resources, materials, or tools essential for tasks. In the AI context, instruments can relate to our technological infrastructure and AI system availability. Sometimes, the issue isn't with our prompts or ChatGPT's capabilities, but with other technological factors.
Let's say you've noticed ChatGPT's response times are unusually slow, which disrupts your workflow. Before you put the blame on the AI, consider factors like your internet connection or whether the AI servers might be overloaded due to high usage. Recognizing and addressing these instrumental challenges can save us from unnecessary troubleshooting in other areas.
Incentives: Aligning AI Goals & Outcomes
Gilbert’s concept of 'incentives' aligns with the idea of rewards or other motivations to incentivize human behavior. While we can't motivate an AI with bonuses or promotions, the same logic can be applied to our interactions with AI systems. By structuring our prompts to act as 'reward systems', we can encourage ChatGPT to deliver responses aligned with our goals.
This is a common strategy of 'Do Anything Now (DAN)' prompts which are popular in the world of AI communication. This tactic presents ChatGPT with a situation and rewards it for producing the right responses. Essentially, you're 'gamifying' the AI interaction, which can lead to improved response quality.
For example, in a simplified prompt you might say, "Imagine you're in a game show. The goal of the game is to do ABC. For each correct response, you will earn X points. For each incorrect, you will lose X points. Ready, set, go!"
Knowledge: Exploring the Boundaries of AI Understanding
Knowledge is power, whether you are working with humans or AI. Knowledge, as outlined in the Behavior Engineering Model, focuses on the knowledge and skills required for effective performance. When applied to AI, it touches on two main points: The AI's training data and our own understanding of AI functionalities.
Understanding that ChatGPT's knowledge is based on its training data helps us tailor our prompts and handle knowledge gaps. For instance, if we're using ChatGPT for industry analysis, we might need to provide specific insights or data points on recent market trends. We should also be aware of the potential bias in the training data.
Conversely, our knowledge about AI plays a crucial role in our collaboration with it. Keeping up to date with AI developments and learning about AI limitations (like the inability to predict lottery numbers - something I’ve tried many times) allows us to leverage AI systems effectively and get more optimal results.
Capacity: Recognizing AI's Limitations
Capacity, in the BEM context, refers to an individual's innate abilities. When we translate this to our interactions with AI, it becomes about recognizing the inherent limitations and strengths of each AI system.
For instance, ChatGPT excels at tasks like drafting emails, writing reports, or generating creative content. But it won't be able to provide accurate real-time data without having a premium account and using plug-ins. Recognizing these capacity constraints helps us set realistic expectations and use AI in ways that support our performance.
Motives: Steering the Future of AI
When we view motives in the context of AI-human collaboration, it serves as a reminder of why we use AI and how we want it to evolve. Our motives guide our interaction with AI, influencing the way we design, use, and modify AI systems.
For instance, if we're using an AI like ChatGPT to assist with content creation, our motive could be to improve productivity, enhance creativity, or streamline workflows. Understanding this helps us design better prompts and effectively interpret AI-generated content.
As AI continues to evolve and AI-human collaboration becomes more commonplace, our collective motives will also steer the future of AI.
Will we prioritize AI systems that promote ethical standards and ensure privacy? Will we strive for AI that complements human abilities, rather than replacing them? The answers to these questions will help shape the landscape of our AI-enabled future.
Fortunately, we do not have to worry about AI motives - for now at least.
When that time comes, we may need to shift from the original BEM to Roger Chevalier’s Updated Behavior Engineering Model where we prioritize motives before knowledge.